Leetcode 973: K Closest Points to Origin
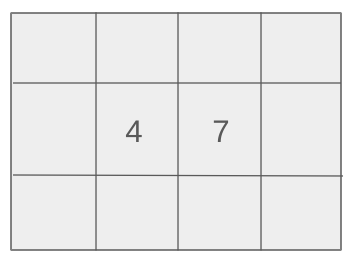
Given an array of points on a 2D plane, find the k closest points to the origin (0, 0) based on Euclidean distance. If multiple points have the same distance, any order is acceptable.
Problem
Approach
Steps
Complexity
Input: An array of points on the X-Y plane and an integer k.
Example: points = [[2, -1], [1, 2], [-3, -4]], k = 2
Constraints:
• 1 <= k <= points.length <= 10^4
• -10^4 <= xi, yi <= 10^4
Output: An array of k points that are closest to the origin.
Example: [[1, 2], [2, -1]]
Constraints:
• The answer may be returned in any order.
Goal: Identify the k points with the smallest Euclidean distances from the origin.
Steps:
• Calculate the Euclidean distance for each point from the origin.
• Sort the points by distance in ascending order.
• Return the first k points.
Goal: Ensure the inputs adhere to the problem's constraints.
Steps:
• 1 <= k <= points.length <= 10^4
• -10^4 <= xi, yi <= 10^4
Assumptions:
• The input points array is valid and non-empty.
• The integer k is always less than or equal to the length of the points array.
• Input: points = [[-1, -1], [2, 2], [4, 5]], k = 1
• Explanation: The closest point is [-1, -1] because it has the smallest Euclidean distance to the origin.
• Input: points = [[2, -1], [1, 2], [-3, -4]], k = 2
• Explanation: The two closest points are [1, 2] and [2, -1] because their distances are smaller than [-3, -4].
Approach: Use a priority queue to efficiently find the k smallest distances.
Observations:
• The problem involves finding the k smallest distances.
• Sorting all distances is inefficient for large inputs.
• Using a priority queue (max-heap) to maintain the k closest points ensures efficient processing.
Steps:
• Define a custom comparator for the priority queue based on distance.
• Iterate through the points, pushing each point into the priority queue.
• If the size of the priority queue exceeds k, remove the farthest point.
• Convert the priority queue to an output array.
Empty Inputs:
• k = 0, no points should be returned.
Large Inputs:
• Maximum constraint of points and k, ensuring efficient handling.
Special Values:
• All points have the same distance to the origin.
Constraints:
• Handle cases where k equals the length of the points array.
vector<vector<int>> kClosest(vector<vector<int>>& pts, int k) {
priority_queue<vector<int>, vector<vector<int>>, cmp> pq;
for(auto it : pts) {
pq.push(it);
}
vector<vector<int>> ans;
while(k--) {
ans.push_back(pq.top());
pq.pop();
}
return ans;
}
1 : Function Definition
vector<vector<int>> kClosest(vector<vector<int>>& pts, int k) {
Defines the function `kClosest`, which takes a 2D vector of points and an integer 'k', and returns the 'k' closest points from the origin.
2 : Priority Queue Declaration
priority_queue<vector<int>, vector<vector<int>>, cmp> pq;
Declares a priority queue `pq` that stores points in a specific order determined by the comparison function `cmp`, allowing efficient extraction of the closest points.
3 : Loop Over Points
for(auto it : pts) {
Iterates through all the points in the input vector `pts` to add them to the priority queue.
4 : Push to Priority Queue
pq.push(it);
Pushes each point from `pts` into the priority queue to sort the points according to the comparison criteria defined by `cmp`.
5 : Answer Vector Declaration
vector<vector<int>> ans;
Declares a 2D vector `ans` to store the closest points as they are extracted from the priority queue.
6 : Extract Closest Points
while(k--) {
Begins a loop to extract the 'k' closest points from the priority queue. This continues until 'k' points are extracted.
7 : Push Closest Point
ans.push_back(pq.top());
Adds the top point from the priority queue (the closest point) to the `ans` vector.
8 : Pop Closest Point
pq.pop();
Removes the top point from the priority queue after it is added to the `ans` vector.
9 : Return Statement
return ans;
Returns the `ans` vector containing the 'k' closest points to the origin.
Best Case: O(n log k)
Average Case: O(n log k)
Worst Case: O(n log k)
Description: Iterate through n points, maintaining a heap of size k.
Best Case: O(k)
Worst Case: O(k)
Description: Heap size is limited to k elements.
LeetCode Solutions Library / DSA Sheets / Course Catalog |
---|
comments powered by Disqus