Leetcode 873: Length of Longest Fibonacci Subsequence
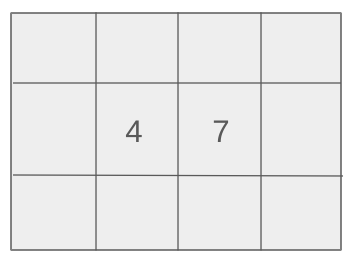
A sequence is called Fibonacci-like if for all indices i, the following condition holds: xi + xi+1 == xi+2. You are given a strictly increasing sequence of positive integers, and your task is to find the length of the longest subsequence that is Fibonacci-like. If no such subsequence exists, return 0.
Problem
Approach
Steps
Complexity
Input: The input consists of a sequence arr of strictly increasing positive integers.
Example: Input: arr = [2, 4, 6, 10, 16, 26, 42]
Constraints:
• 3 <= arr.length <= 1000
• 1 <= arr[i] < arr[i + 1] <= 10^9
Output: Return the length of the longest Fibonacci-like subsequence. If no such subsequence exists, return 0.
Example: Output: 6
Constraints:
• The output should be a single integer indicating the length of the longest Fibonacci-like subsequence.
Goal: The goal is to find the longest subsequence of arr that satisfies the Fibonacci-like property.
Steps:
• Iterate over all pairs of elements arr[i] and arr[j], with i < j, and try to extend the sequence by checking if arr[i] + arr[j] exists in arr.
• Track the length of the Fibonacci-like subsequences found using dynamic programming or hash-based methods.
• Return the length of the longest Fibonacci-like subsequence.
Goal: The input sequence is strictly increasing, and the elements lie within the specified range.
Steps:
• 3 <= arr.length <= 1000
• 1 <= arr[i] < arr[i + 1] <= 10^9
Assumptions:
• The input array is strictly increasing.
• A subsequence is obtained by removing some or none of the elements from the original array while maintaining the relative order.
• Input: Input: arr = [2, 4, 6, 10, 16, 26, 42]
• Explanation: The longest Fibonacci-like subsequence is [2, 4, 6, 10, 16, 26] which has length 6.
• Input: Input: arr = [1, 2, 3, 5, 8, 13, 21]
• Explanation: The longest Fibonacci-like subsequence is [1, 2, 3, 5, 8, 13] which has length 6.
Approach: We will utilize dynamic programming and hash sets to efficiently track and extend possible Fibonacci-like subsequences.
Observations:
• We need to check all pairs (i, j) where i < j, then check if arr[i] + arr[j] is also present in arr.
• By using a hash set for fast lookups, we can avoid checking each possible subsequence manually.
• Dynamic programming can help track the lengths of valid Fibonacci-like subsequences efficiently.
Steps:
• Create a hash set to store the elements of arr for fast lookups.
• For each pair (arr[i], arr[j]) with i < j, initialize a variable to track the length of the subsequence and try to extend it by checking if arr[i] + arr[j] exists in the set.
• Store the length of the longest subsequence found during the iteration and return it.
Empty Inputs:
• If the input array has fewer than 3 elements, return 0, as no Fibonacci-like subsequence can be formed.
Large Inputs:
• For large inputs (with up to 1000 elements), the solution should be efficient enough to handle the worst-case scenario within the time limits.
Special Values:
• The array may contain elements with values up to 10^9, so we need to ensure efficient lookups and handling of large numbers.
Constraints:
• The solution must handle inputs with up to 1000 elements and values up to 10^9.
int lenLongestFibSubseq(vector<int>& arr) {
unordered_set<int> s(arr.begin(), arr.end());
int res = 2, n = arr.size();
for(int i = 0; i < n; i++) {
for(int j = i + 1; j < n; j++) {
int a = arr[i], b = arr[j], l = 2;
while(s.count(a + b))
b = a + b, a = b - a, l++;
res = max(res, l);
}
}
return res > 2? res: 0;
}
1 : Function Definition
int lenLongestFibSubseq(vector<int>& arr) {
The function definition starts by accepting an integer vector `arr`, which represents the input sequence to be processed.
2 : Data Structures
unordered_set<int> s(arr.begin(), arr.end());
An unordered set `s` is created from the elements of the array `arr` for constant time lookup during the subsequence search.
3 : Initialization
int res = 2, n = arr.size();
Initializes the result `res` to 2 (the minimum length of a Fibonacci-like subsequence) and calculates the size `n` of the input array `arr`.
4 : Loop
for(int i = 0; i < n; i++) {
The outer loop starts, iterating through each element `arr[i]` in the array.
5 : Nested Loop
for(int j = i + 1; j < n; j++) {
The inner loop starts, iterating over the elements after `arr[i]`, creating all pairs for potential Fibonacci subsequences.
6 : Variable Assignment
int a = arr[i], b = arr[j], l = 2;
Assigns the values of `arr[i]` and `arr[j]` to `a` and `b`, and initializes the length of the current Fibonacci-like subsequence `l` to 2.
7 : While Loop
while(s.count(a + b))
Checks if the sum of `a` and `b` exists in the set `s`. If it does, it continues to build the subsequence.
8 : Update Values
b = a + b, a = b - a, l++;
Updates the values of `a` and `b` to continue forming the subsequence and increments the subsequence length `l`.
9 : Update Result
res = max(res, l);
Updates the result `res` with the maximum length of the Fibonacci-like subsequence found so far.
10 : Return Statement
return res > 2? res: 0;
Returns the result `res` if it is greater than 2 (valid Fibonacci-like subsequence), otherwise returns 0.
Best Case: O(n^2)
Average Case: O(n^2)
Worst Case: O(n^2)
Description: The time complexity is quadratic due to the nested iteration over all pairs of elements.
Best Case: O(n)
Worst Case: O(n)
Description: The space complexity is linear due to the space required for storing the elements of the array and any intermediate results.
LeetCode Solutions Library / DSA Sheets / Course Catalog |
---|
comments powered by Disqus